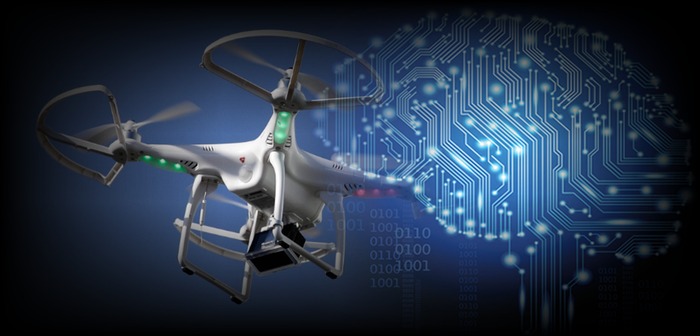
Swift AI employed a method called deep reinforcement learning to secure victory in 15 out of 25 races against world champions
After dominating humans in activities ranging from chess and Go to StarCraft and Gran Turismo, artificial intelligence (AI) has taken on a new challenge and triumphed over world champions in an actual sport.
The most recent individuals to experience the sting of defeat by AI are three skilled drone racers who were surpassed by an algorithm that learned how to navigate a 3D racecourse with a drone at high speeds, all while minimizing crashes—well, at least reducing them significantly.
Created by researchers at the University of Zurich, Swift AI emerged victorious in 15 out of 25 races against world champions and achieved the fastest lap time on a course where drones can reach speeds of up to 50mph (80km/h) and endure accelerations of up to 5g, an intensity that can cause some individuals to lose consciousness.
Elia Kaufmann, a researcher involved in Swift’s development, remarked, “Our achievement represents the inaugural instance where an AI-driven robot has defeated a human champion in a physical sport crafted by and for humans.”
In first-person view drone racing, participants fly drones through a course marked with gates that must be navigated cleanly to avoid collisions. These pilots perceive the course through a video feed transmitted from a camera mounted on the drone.
In their article published in Nature, Kaufmann and his colleagues detail a series of one-on-one races pitting Swift against three champion drone racers: Thomas Bitmatta, Marvin Schäpper, and Alex Vanover. Prior to the competition, the human pilots had a week to practice on the course, while Swift underwent training in a simulated environment featuring a virtual replica of the racecourse.
Swift utilized a technique known as deep reinforcement learning to determine the optimal commands for swiftly navigating the circuit. As this approach involves trial and error, the drone experienced numerous crashes during training, but since it was a simulation, the researchers could easily restart the process.
During a race, Swift transmits video footage from the drone’s built-in camera to a neural network responsible for identifying the racing gates. This data is combined with inputs from an inertial sensor to estimate the drone’s position, orientation, and velocity. These estimations are then used as input for a second neural network, which determines the appropriate commands to be sent to the drone.
Race analysis revealed that Swift consistently exhibited faster starts and executed tighter turns compared to human pilots. Swift’s quickest lap was completed in 17.47 seconds, half a second faster than the fastest human pilot. However, Swift was not flawless; it lost 40% of its races against humans and experienced several crashes. It appeared that the drone was sensitive to environmental factors like changes in lighting.
The world champions had mixed emotions following these races. Thomas Bitmatta expressed, “This marks the beginning of something that could revolutionize the entire world. On the other hand, I’m a racer, and I don’t want anything to outpace me.” Marvin Schäpper noted, “Racing against a machine feels different because you know that the machine never gets fatigued.”
A significant breakthrough lies in Swift’s ability to tackle real-world challenges like aerodynamic turbulence, camera blurring, and variations in lighting conditions, issues that can confound systems relying on pre-calculated flight paths. Kaufmann mentioned that this same approach could prove valuable for tasks such as drones searching for individuals in burning buildings or conducting inspections of large structures like ships.
While the military maintains a keen interest in AI-powered drones, there remains skepticism that this recent development would have substantial implications for warfare. Dr. Elliot Winter, a senior lecturer in international law at Newcastle Law School, cautioned against assuming that advancements like these can readily translate into a military context for use in military drones or autonomous weapon systems, particularly those involved in critical processes like target selection.
Alan Winfield, a professor specializing in robot ethics, acknowledged that AI has “inescapable” applications in the military but expressed uncertainty regarding how the recent developments could provide significant military advantages, apart from the potential use of drone formations closely following aircraft.
Kaufmann shared a similar skepticism, stating, “Nearly all drones are deployed in expansive battlefield scenarios, primarily for reconnaissance or as weapons against slow-moving and stationary targets.